Articles of author kemtai
July 16, 2024
1 min reading

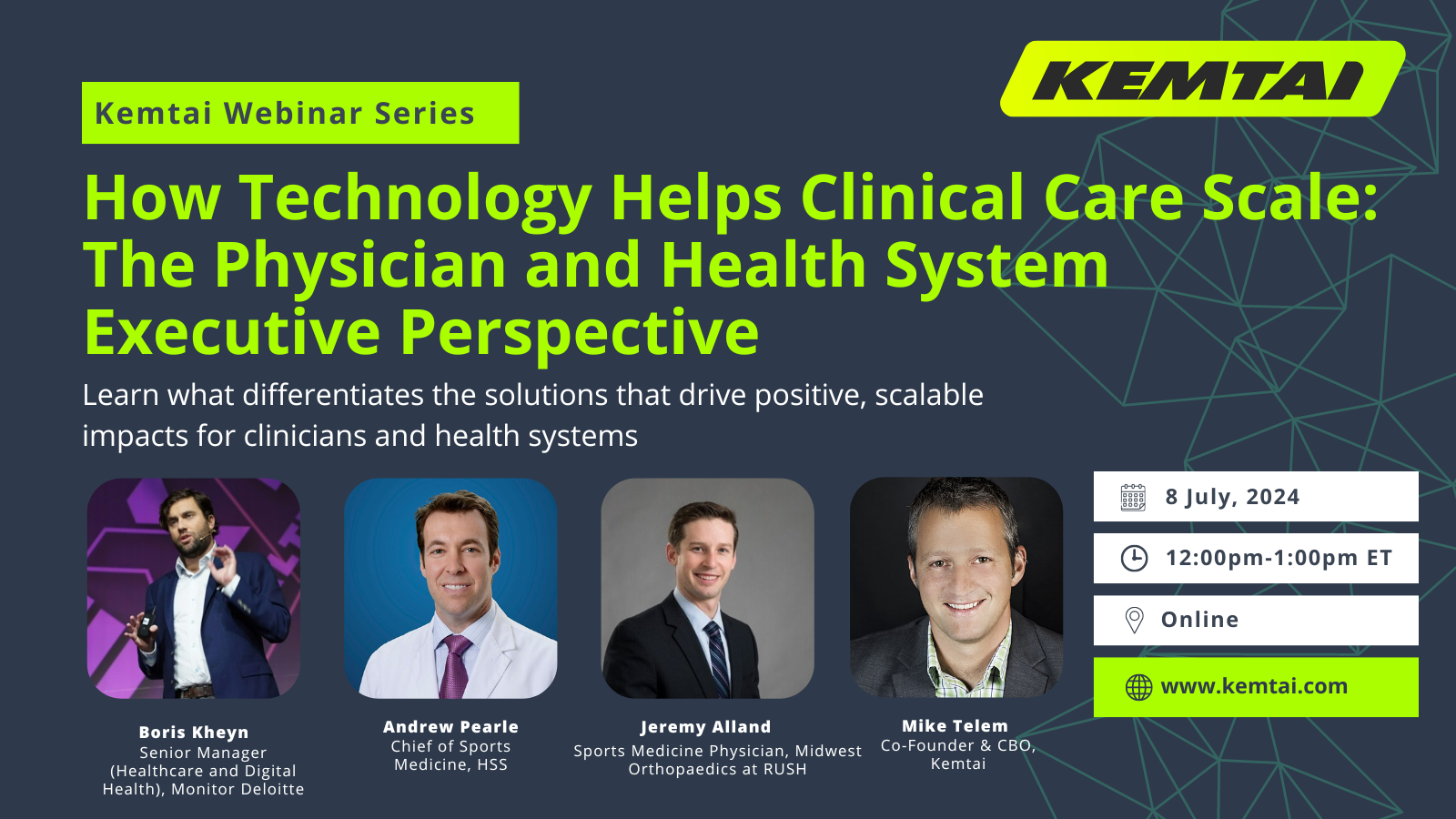
Kemtai Summer Webinar Series 2024
July 15, 2024
6 min reading

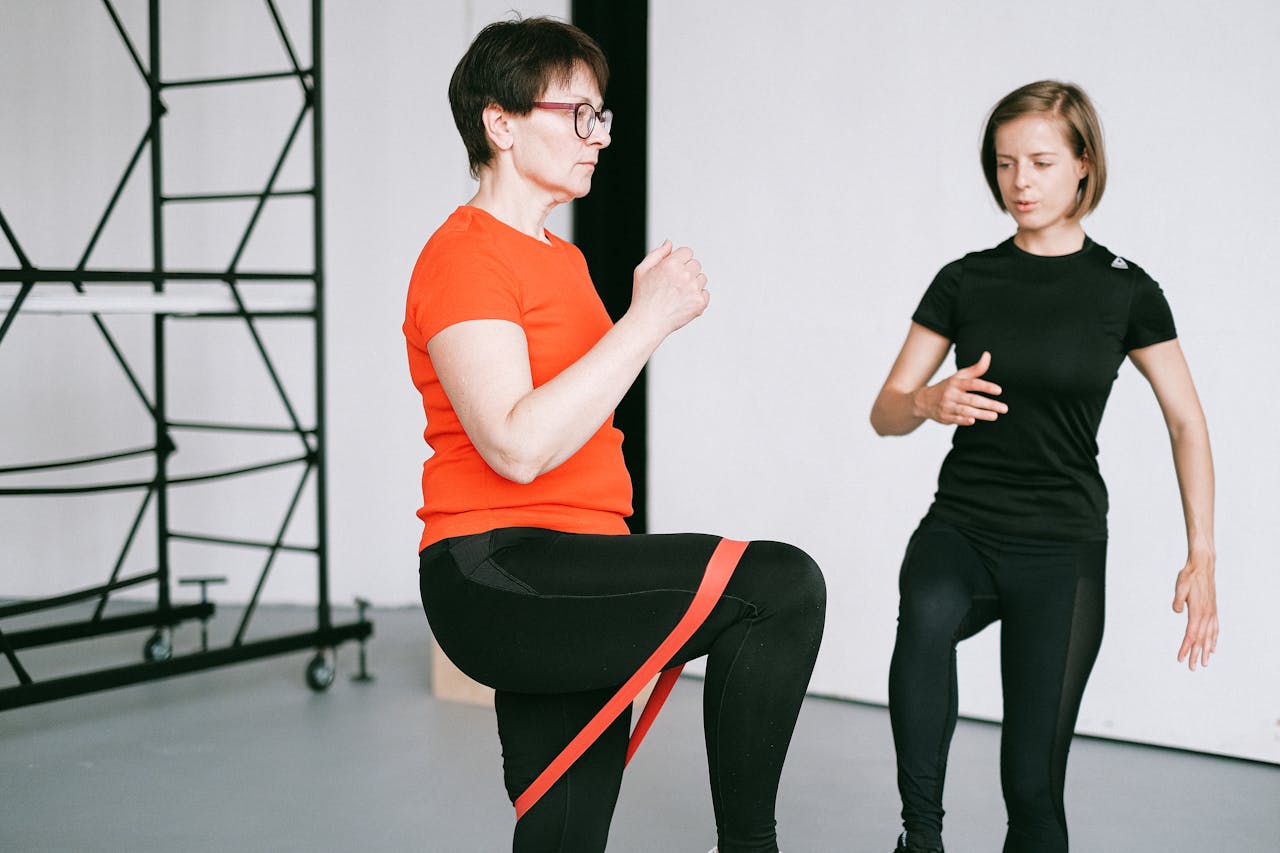
Physiotherapy vs. Surgical Treatment in Musculoskeletal Disorders: An In-Depth Comparison
July 2, 2024
3 min reading

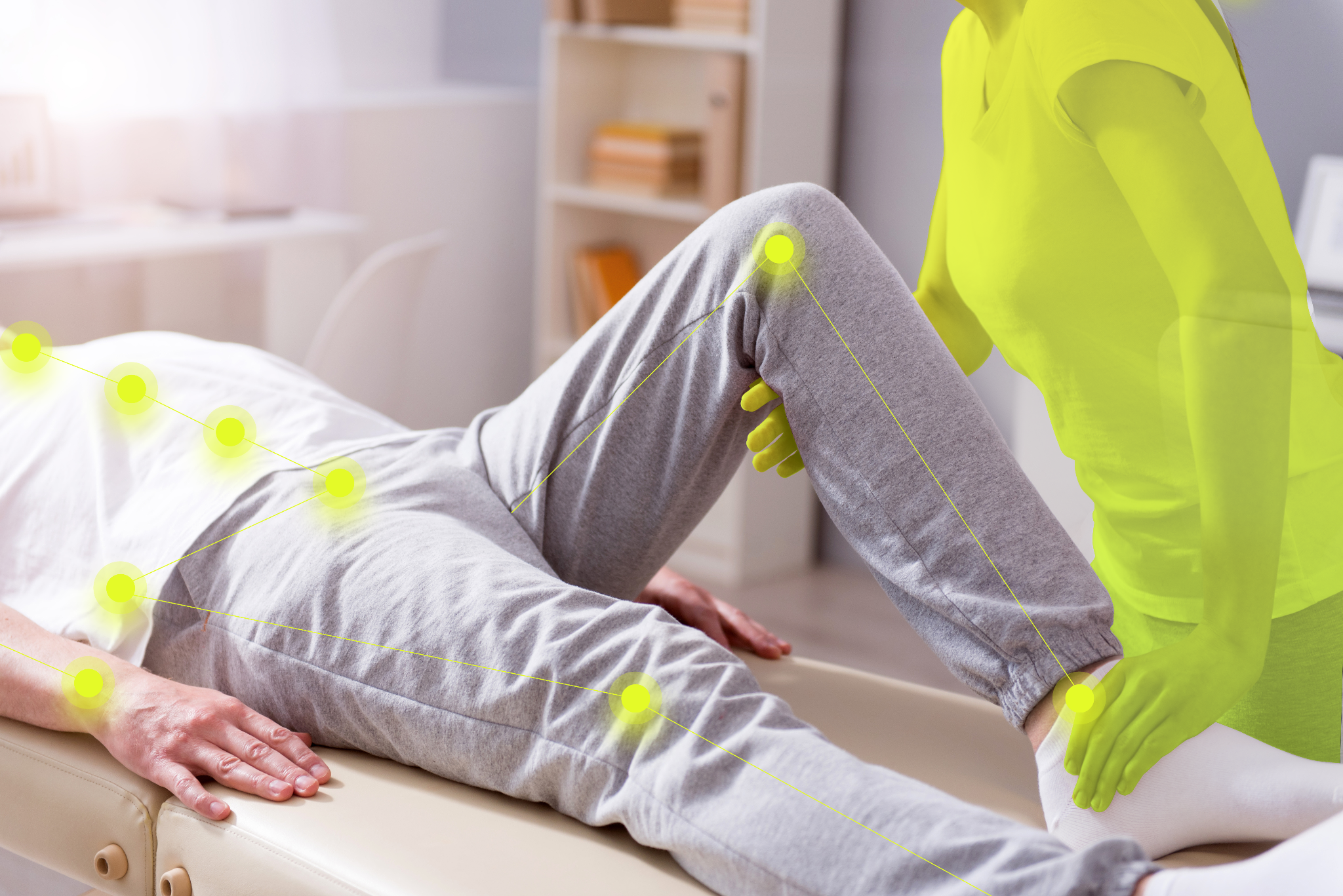
PHTI Report Demonstrates that Virtual MSK Solutions Positively Impact Outcomes and Financials
June 5, 2024
6 min reading

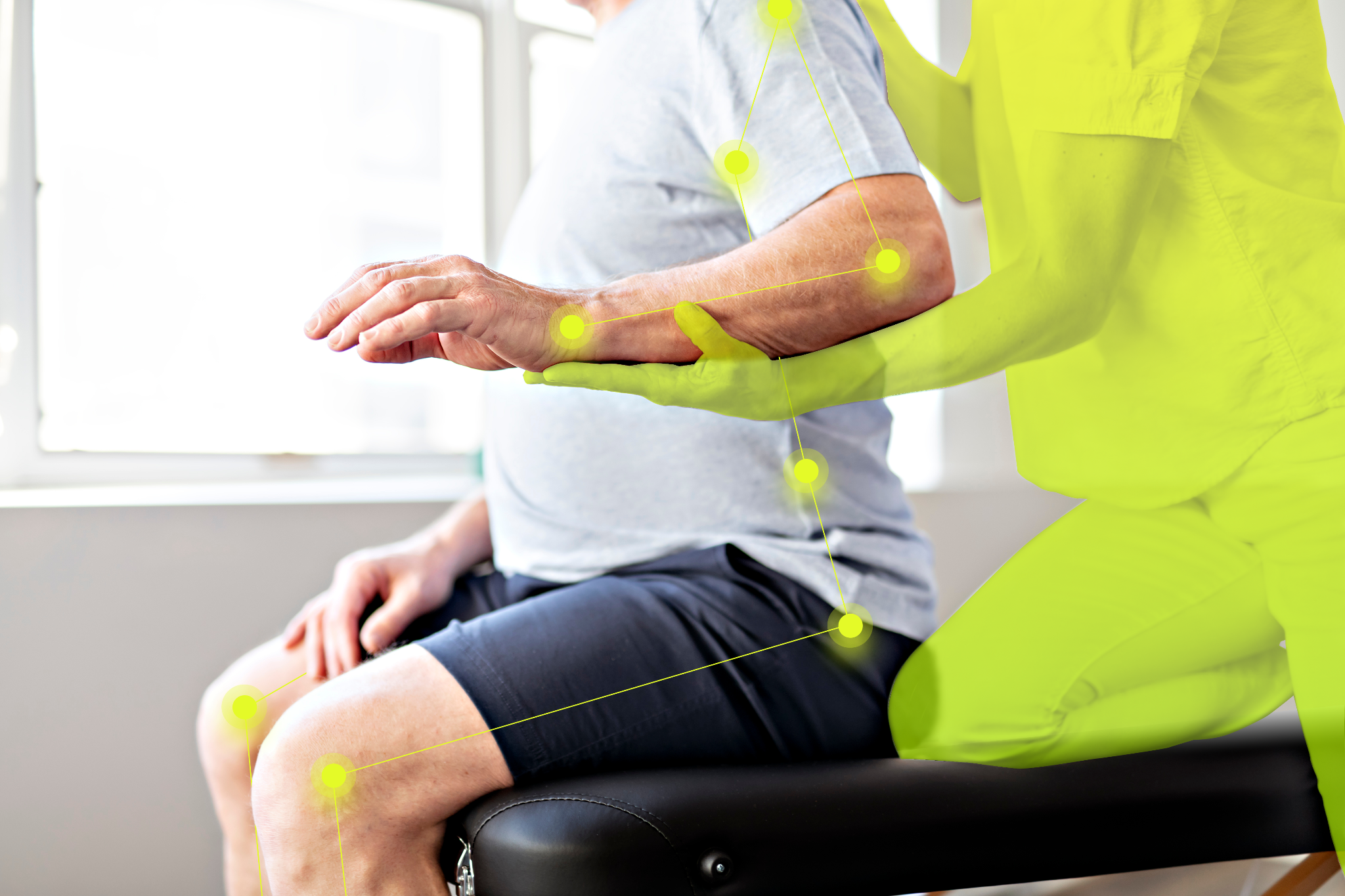
The Benefits of Computer Vision for Orthopedic Rehabilitation
May 2, 2024
5 min reading

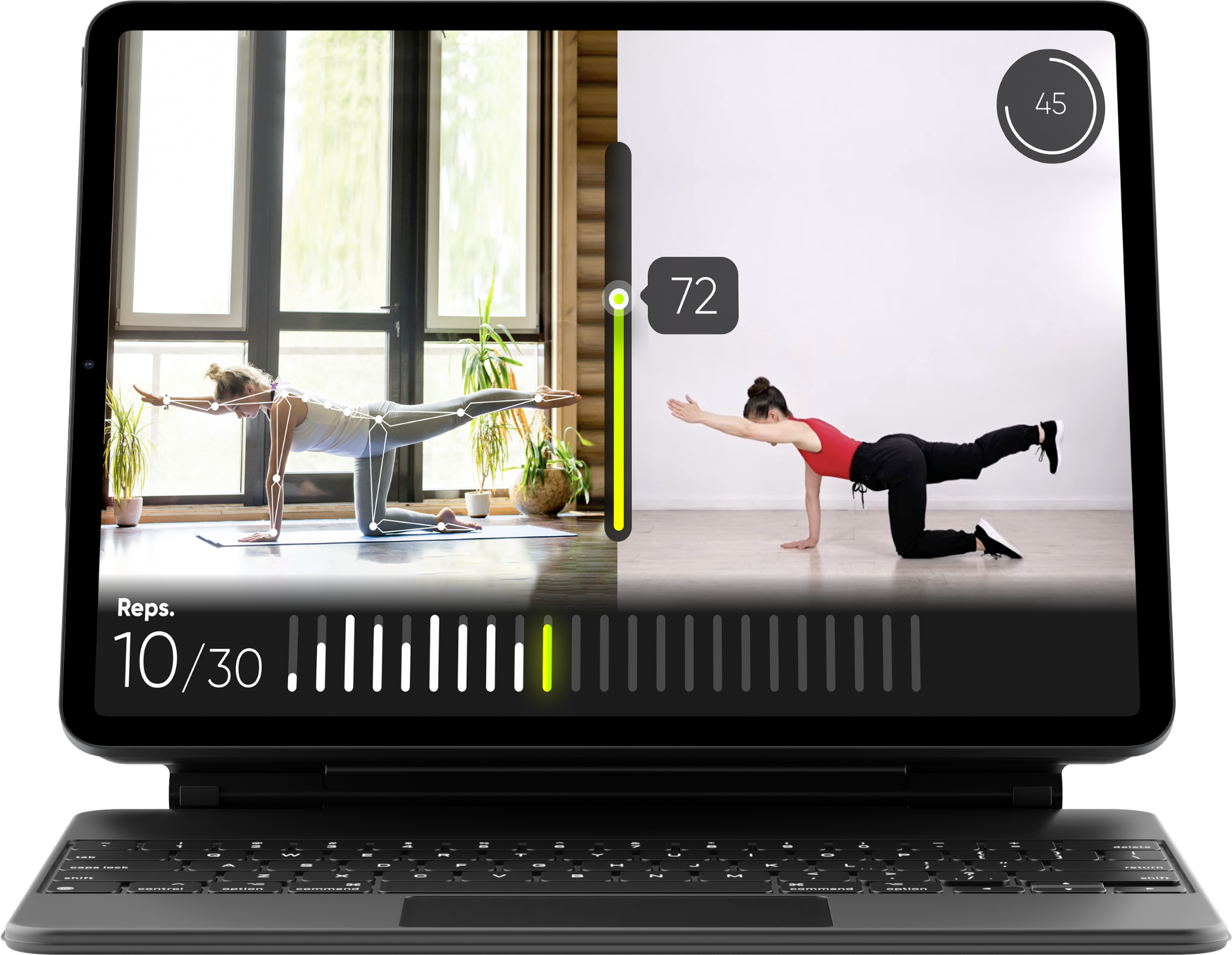
Kemtai drives 72% Average Adherence in Cross-Industry Pilots
May 1, 2024
5 min reading

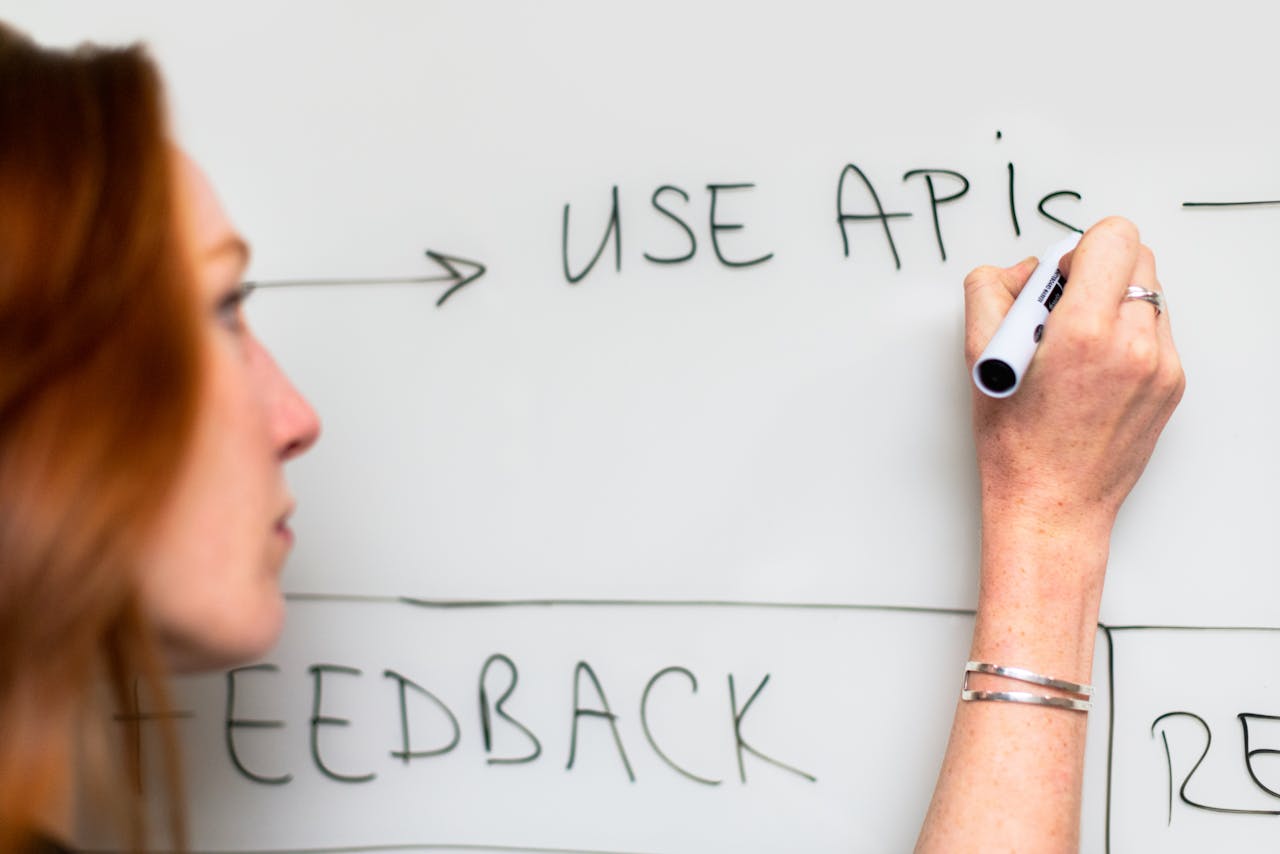
Build vs. Buy Part III: Buying the Right Computer Vision Technology
April 18, 2024
6 min reading

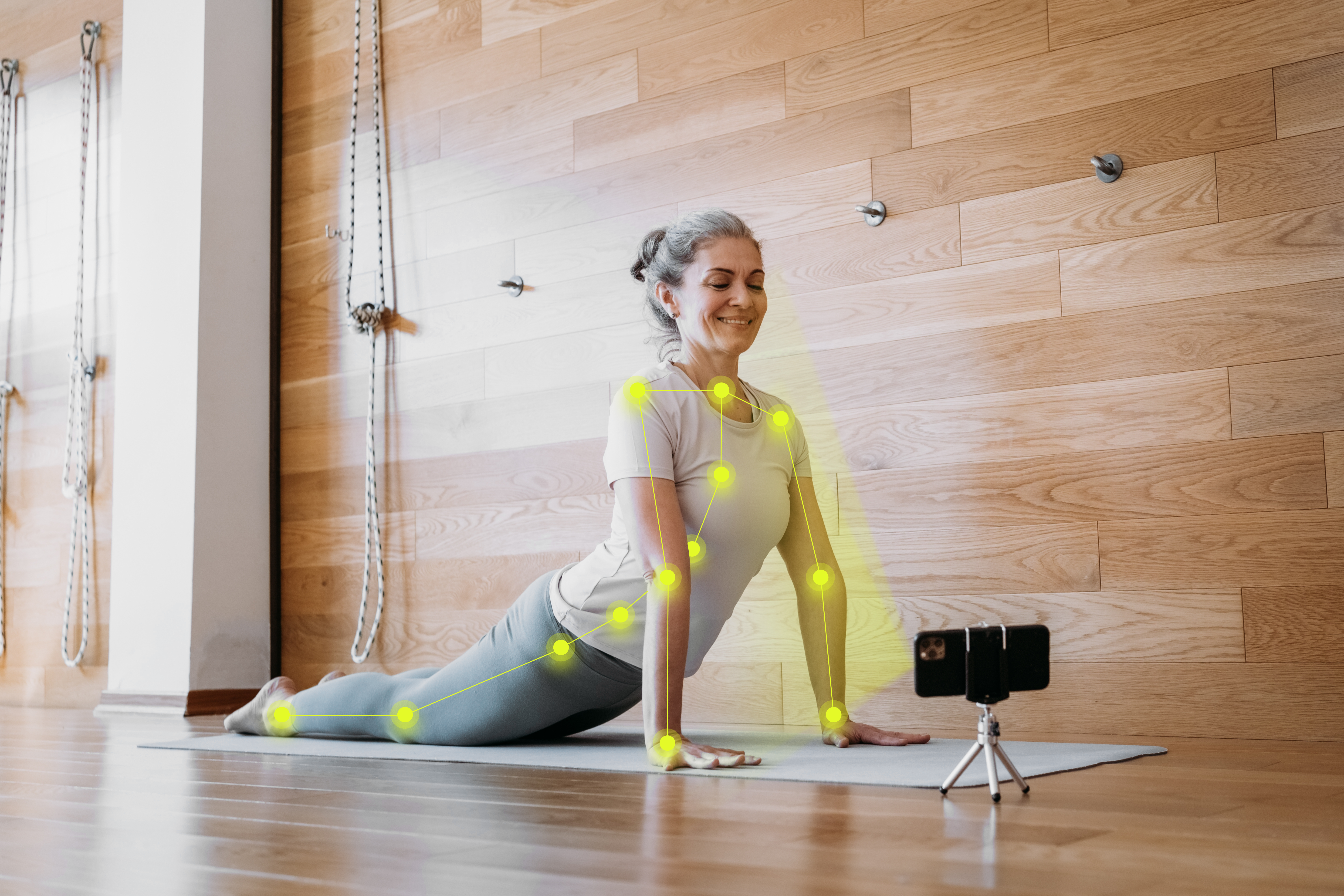